Article by Irina Steenbeek, Managing Director, Data Crossroads.

We, data management professionals, often use the term “data management framework.” But do we all have the same understanding of its meaning and the various approaches to its implementation?
In this article, we will
– Discuss existing data management frameworks
– Present the utilization statistics of various frameworks
– Define the term “data management framework”
– Illustrate a common approach to establishing a data management framework
Hear more from Irina at the Data Governance Conference and Master Data Management Summit, 16 – 19 May, 2022. View the full agenda and find out more here; https://bit.ly/3L6Vefm
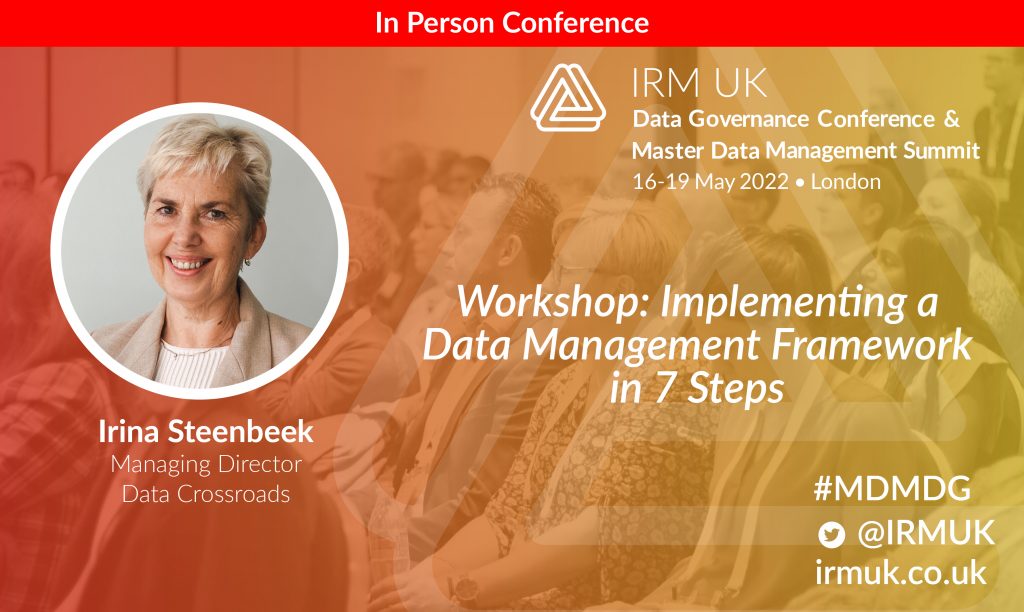
Existing data management frameworks
Both the DAMA Guide to the Data Management Body of Knowledge and DCAMTM (The Data Management Capability Assessment Model) define themselves as data management frameworks (DMF). Let’s briefly explore their respective understandings of what a data management framework is.
DAMA-DMBOK 2
DAMA-DMBOK2 stipulates that “the DAMA Data Management Framework […] provides the context for work carried out by data management professionals within various Data Management Knowledge Areas.” My primary concern about this definition is the following: it is not only data management professionals who carry out work in the data management area; business professionals are also involved.
DAMA-DMBOK2 defines three key diagrams used to visualize its framework: “The DAMA Wheel, The Environmental Factors hexagon, The Knowledge Area Context Diagram.” We know that DAMA-DMBOK2 identifies 11 Knowledge Areas that constitute data management. The challenge is that these knowledge areas are logically connected and interrelated with each other. A company needs to take these dependencies into consideration when planning and scoping the implementation of a data management framework. You can’t implement data quality without implementing several other data management capabilities such as data governance, data modeling, information systems architecture, and so on. The following statement: “None of the pieces of the existing DAMA Data Management framework describe the relationships between the different Knowledge Area” completely devalues the practical application of DAMA-DMBOK2.
DCAMTM
While DAMA-DMBOK2 is a publicly available source, DCAMTM is closed for public access: only Enterprise Data Management Council members have access to this guide. Recently, EDC made the “Cloud Data Management Capabilities Framework” available for free download. This document discloses the approach EDM Council takes to defining the framework. First of all, the EDM Council framework is Data Management Capability Assessment Framework. It describes data management in terms of different capabilities having different maturity levels. The interpretation of a business capability differs significantly from a term used by The Open Group. If even the EDM Council framework has a practical and operational focus, it still lacks an integrated viewpoint on the implementation of data management.
These two frameworks have conceptual differences that are illustrated in Table 1.

Table 1: Conceptual differences between DAMA-DMBOK2 and DCAMTM data management frameworks.
The nature of data management building blocks can vary greatly. DAMA-DMBOK2 operates with Knowledge Areas, while DCAMTM uses components/capabilities/sub-capabilities. The number of building blocks and their content can also have remarkable differences. Furthermore, even building blocks with the same name can have significant differences in their content and deliverables. I mapped these two guides and described the differences between their models in the series of articles: “DAMA-DMBOK2 vs DCAM® .2- which framework to choose?”
In practice, these differences mean the following: two companies using these two different guidelines will have different forms of data management, and their data management frameworks will be incomparable.
In my opinion, these two frameworks share another similar shortcoming: they don’t consider the dynamic nature of data that is expressed by the concept of a data lifecycle and, therefore, realized in multiple data chains.
Usage statistics of various frameworks
I ran the same poll on LinkedIn twice- one year apart. Figure 1 shows the results of the poll.

Figure 1: The utilization of various data management frameworks.
Based on the results, I drew the “obvious” conclusion that DAMA-DMBOK2 can be considered the leading data management framework. Remarkably, 25% of respondents claimed having developed new or adjusted existed frameworks. After interviewing the respondents, I realized that my conclusions were not entirely accurate. Respondents that use DAMA-DMBOK2 still make significant adjustments to this framework to tailor it to a company’s practices. So, it seems that every company that implements a data management framework should to some degree “reinventing the wheel.”
Data management framework: what and why?
Every company manages data in one way or another. The implementation of data management (DM) means establishing a data management function as a part of a company’s organizational structure. In order to do this, the company needs to develop and implement a data management framework. In this context, a data management framework (DMF) is a collection of interrelated components that shape data management into a business function. A DMF consists of numerous components and serves a variety of purposes, as shown in Figure 2:
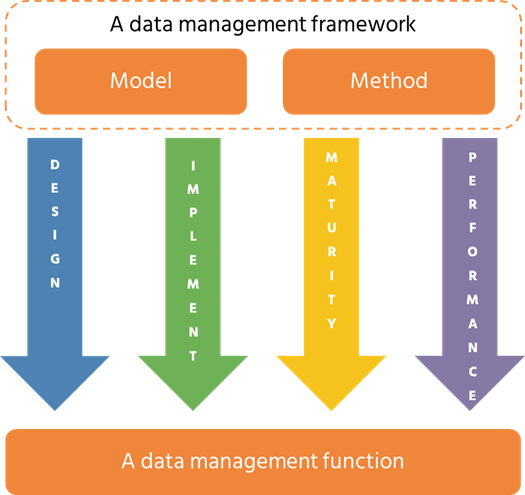
Figure 2: The definition of a data management framework.
The key components are models and methods. A set of data management capabilities is an example of a model. A method is an explanation or guideline on how to do something. In this respect, all documentation related to a data management framework such as policies, standards, and so on, are examples of methods.
A data management framework serves four purposes: design, implement, measure maturity, and assess the performance of a data management function.
We can describe and define data management and a data management function at various organizational levels: strategic, operational, and functional. A data management framework should provide different models and methods depending on the organizational level it is applied to. Table 2 illustrates the content of the “Orange” data management framework by Data Crossroads.

Table 2: The content of the “Orange” data management framework by organizational level.
I described this framework in the series of articles: “The ‘Orange’ Model of Data Management.” For more detail, you can consult my new book “The ‘Orange’ Data Management Framework: Design, Implement, and Measurement of Maturity and Performance. A Practitioner’s Guide,” that will be published in May-2022.
In this book we answered two questions: what is a data management framework, and why does a company needs one. Let’s explore a method of implementation.
Data management framework: how?
Regardless of the chosen data management framework, a company should perform similar steps to establish a data management function. Figure 3 identifies these steps:

Figure 3: The 7-step method to establishing a data management function.
These steps assume that a chosen data management framework can assist in designing, implementing, and measuring the maturity and performance of a data management function.
Step 1: Scoping a data management framework and a data management function
The scope of the required data management function varies by company and is dependent on many factors. The key rule is that the defined scope should meet a company’s needs and resources. The factors that a company should consider when establishing a formal data management function are:
• Business drivers
A business driver is a reason for a company to start such an initiative. A company should take into account business factors from both external and internal environments.
The most common drivers are various regulations (i.e., GDRP) and business change (i.e., digital transformation, the implementation of AI/ML solutions to improve customer experience).
• Needs of various stakeholders
• Business drivers identify stakeholders. Stakeholders can be external and internal. Different stakeholders will have diverse, sometimes controversial, needs and expectations from a data management initiative. Assume a company decides to start a digital transformation solution. It is impossible to digitalize all business processes at once as significant investment will be required. Financial and commercial departments will have different motivations. The financial department expects to improve efficiency and productivity while the commercial department focuses on revenue growth by improving the customer experience.
• “Enterprise” scope
A company can start implementing a data management function across the whole organization or only for certain business units. The best approach depends on the chosen business driver.
• Set of required data management capabilities
Data management is a multifunctional discipline. To meet the requirements of a specific driver, a company may need different capabilities. Common basic data management capabilities are data governance, data modeling, data and application architecture, and data quality.
Step 2: Performing a preliminary maturity assessment
Every company manages data in one way or another. This means that some data management capabilities already exist within the company, even if it does not have a formal data management function in place. A preliminary maturity assessment allows for the assessment of existing capabilities and identification of potential gaps.
Step 3: Designing a data (management) strategy and roadmap
Based on the results of the gap analysis in step 2, a company should identify its strategic vision for data management in the medium- or long-term. The “Orange” DMF identifies a strategy and roadmap as planning documents.
Step 4: Designing data management capabilities
Depending on the chosen framework, a company should design the required capabilities. The “Orange” DMF defines a capability as a company’s ability to reach goals or deliver outcomes. Six dimensions constitute each data management capability, as shown in Figure 4.

Figure 4: Six dimensions of a data management capability.
The design starts with the identification of required deliverables. A company should have policies, standards, and other regulatory documents to produce deliverables. Processes assist in adhering to regulations. Roles are required to perform processes. Tools enable processes. And, of course, a company must have a budget to establish a capability.
Step 5: Implementing a data management framework
The best approach to implementing a data management framework varies by company and depends on the company’s business driver, size, and resources available. The “Orange” DMF identifies three key approaches: centralized, decentralized, and hybrid. Each of these approaches has its advantages and disadvantages and is applicable in different circumstances.
Step 6: Performing a detailed maturity assessment
A company should perform a detailed maturity assessment at the granular level of capability dimensions and items, for example, at the level of processes, policies, and so on. This approach allows for the development of detailed short-term planning. It also assists in establishing a system of key performance indicators (KPI).
Step 7: Setting up a system of KPIs
A company should set up two types of KPIs: one to measure the progress in establishing a data management capability and another to measure the efficiency of operations. KPIs can be set up at various abstraction levels.
I want to conclude this article by assessing the ability of each of the data management frameworks we’ve discussed to serve the following four purposes: design, implement, measure maturity, and measure performance of a data management function. In Table 3, I share my assessment of the three frameworks:

Table 3: The comparison of three frameworks.
Each of these three frameworks can be used to design a data management framework. Both DAMA-DMBOK2 and DCAMTM 2.2 lack an integrated implementation approach. While DAMA-DMBOK2 provides guidelines regarding maturity assessment, DCAMTM 2.2 was initially developed as a maturity assessment methodology. DAMA-DMBOK2 provides metrics per Knowledge Area while DCAMTM 2.2 allows for the creation of such metrics.
Should you have other opinions on what we’ve discussed in this article, I would much appreciate getting your feedback.
This article has been originally published at the Data Crossroads site.