What would it be like to use an unbiased algorithm?
An insurance scoring application could assign coverage and costs quite randomly, having no bias towards good drivers with clean records. Or perhaps facial recognition would open your laptop to everyone, having no preference in favor of owners or account users or, indeed, no bias towards faces rather than hands or feet.
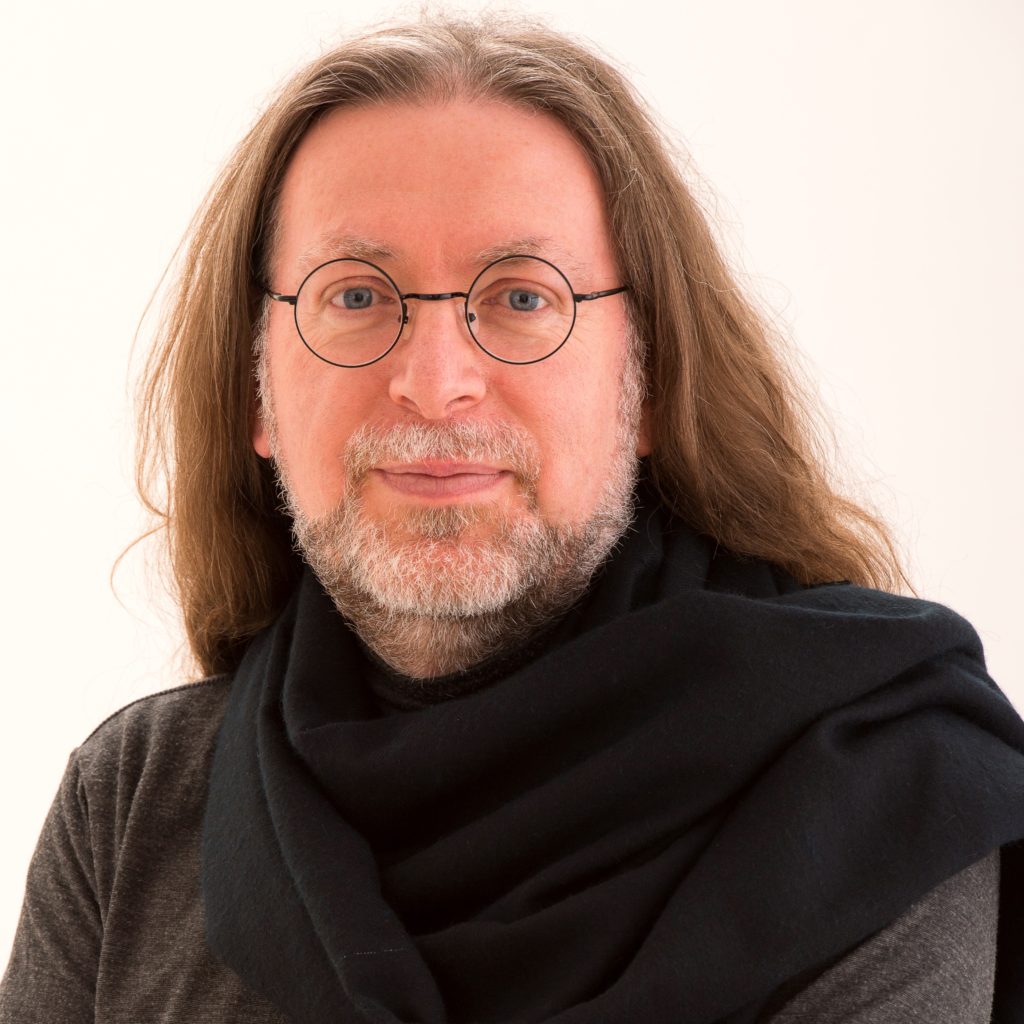
Donald Farmer, Principal, TreeHive Strategy
Donald will be co-chairing and speaking at the Business Intelligence and Analytics & Enterprise Data Conference Europe 18-22 November 2019, London.
He will be speaking on the subject, ‘The New Boundaries of Business Intelligence‘ and will be presenting the one-day course ‘The Analytic User Experience as a part of Data Ed Week Europe 2019
The very purpose of machine learning is to encode statistical biases. An algorithm without partiality is hardly an algorithm at all.
But of course we use the term bias somewhat casually to mean unfair preferences or prejudices we don’t agree with.
Our challenge then is not so much to eliminate bias from algorithms, but to define our preferences clearly enough that they can specified in advance by project managers, designers or data scientists and then tested for by QA teams.
As a good practice in general, I suggest that specifications for software products and services (including machine learning projects) should include both measures of success and non-goals. The latter are definitions of what is out of scope. For example, a text generation system may output only English by design, so generating other languages would fall under non-goals.
Increasingly, I suggest that machine learning projects should include negative measures of success. These are tricky to define, but could include, for example, that a system would have no detectable bias against identified minorities. Defining these intentions first has advantages: for everyone from data engineers and data scientists to developers and QA, it draws their attention to the need for data sets, algorithms and even UX to meet that standard.
I see too many projects with poorly defined measures of success, few if any non-goals and rarely any negative measures.
Some people have disapproved of calling non-bias a negative goal, the objection being more to the tone it sets than the practical implication. It’s not negative to want to reduce prejudice.
But to be clear, for me a negative goal represents a crucial contrast to positive goals: the phrasing intentionally indicates something the system must not do. Designing and testing specifically to catch such errors requires both a new mindset and often different techniques.
In short, we cannot remove bias from machine learning, but we can be (and must be) explicit about the preferences we seek to optimize and the prejudices we want to undo.
Donald Farmer is an internationally respected speaker and writer, with over 30 years’ experience in data management and analytics. His background is very diverse, having applied data analysis techniques in scenarios ranging from fish-farming to archaeology. He worked in award-winning start-ups in the UK and Iceland and spent 15 years at Microsoft and at Qlik leading teams designing and developing new enterprise capabilities in data integration, data mining, self-service analytics, and visualization. Donald is an advisor to globally diverse academic boards, government agencies, and investment funds and also advises several start-ups worldwide on data and innovation strategy.
Copyright Donald Farmer, Principal, TreeHive Strategy