In Gartner’s latest “Hype Cycle for Data Management”, MDM is positioned in “the trough of disillusionment” phase, the phase that follows the initial positive hype and the following “peak of inflated expectations” phases. According to Gartner, with some exceptions, technologies traverse its “hype cycle” at different speeds, with “fast-track” technologies taking two to four years and “long-fuse” ones taking one or two decades. Given its origins in the mid-2000s, MDM seems to fall into the “long-fuse” group, though not exhibiting many of the indicators ascribed to the group.
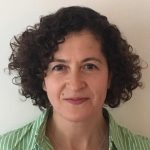
Yasemin Kural, Principal Data Architect, Farfetch
Yasemin will be speaking at the IRM UK Enterprise Data & Business Intelligence and Analytics Conference Europe 18-22 November 2019, London.
She will be speaking on the subject ‘Where’s Wally? Unearthing & Aligning MDM Efforts at Farfetch‘
According to Gartner’s 2017 report, the MDM market grew 4.9% in 2016, lagging behind the overall software market and achieving only a fraction of the 24% growth it achieved from 2007 to 2008. Gartner concluded that growth in the MDM market was “being tempered by alternative technologies that meet customer needs”. Some analysts list data science and graph databases amongst such new technologies. Gartner has furthermore suggested in their 2018 MDM Magic Quadrant report that the “always-complex” MDM market was showing a share shift from larger vendors to smaller vendors that are introducing innovative approaches to MDM.
The inherent complexities of the MDM market, emergence of alternative technologies and efforts by the MDM vendors to re-brand their offerings to capture the zeitgeist are all making it confusing for the potential customers to identify products that can address their major data challenges. Surprisingly, this seems to happen even at the technology category level, where distinctions are relatively clear, e.g. when choosing between Data Quality vs. MDM vs Data Governance tools. The situation is further exacerbated when vendors claim that their offering(s) have capabilities to address data management needs that are typical use cases for a different family of tools.
Let’s look a bit closer at the “new technologies” and “innovative approaches” that are blamed for stealing the show. It would be more appropriate to look at graph databases as complementary rather than competing with MDM as MDM is not just a means of creating flexible data models and storing data. The challenge from Data Science is more pressing, though arguably also misplaced.
Data Science is tasked with uncovering truths based on analysis of an organisation’s data, but it is not a panacea for all the data needs and pain areas a typical organization faces. In particular, it’s inconceivable to see it as an alternative to analytical MDM. It has nothing to say about and no way to represent the vision/strategy of an organization. It cannot generate product hierarchies/categories for planned sales as they cannot be just derived from existing data; it cannot define sales territories for a new product line as these are often the product of complex and pragmatic decisions; likewise, it would be spurious to expect Data Science to identify geographic entities/borders, or harmonize data across different coding schemes. These are all examples of classifications that subject matter experts collaboratively generate and maintain to achieve a shared understanding of their business eco-system.
In short, analytical MDM is a top-down view of the business as it performs or is expected to perform; it exists in some preliminary form even before there is any data sets for Data Science to wrangle. Operational MDM is a bottom-up view of the business activities and measurements as they happen, and is typically a bit out of date compared to the analytical view, as it cannot quickly keep up with the changes in business environment and strategy. Data Science can complement the picture by revealing whether the vision of the business leaders fits in with the reality. Likewise, analytical MDM can be utilised to validate and make sense of Data Science discoveries.
Yet, it is undeniable that Data Science is the new kid in the block, and MDM has lost some of the sheen from its early days. Nowadays, not many CDOs can expect to wow their board by championing a new MDM initiative, and some are even sceptic about its potential and relevance themselves. It may be different for well-defined problems with clear business cases, e.g. Product Information Management (PIM) initiatives for CPG companies or data lineage/governance requirements for heavily regulated industries like finance and pharmaceuticals. However, it is often tricky to get traction for organization-wide analytical MDM initiatives, not only because of the tendency to see MDM as yesterday’s technology but also due to lack of experience in making the business case, perceived costs of MDM initiatives and the typically small resource pools available for specific MDM technologies.
This is unhelpful because MDM is not a fad, but a discipline that is critical for providing business users with actionable insights. What’s more, it is pervasive; if it is not done transparently and collaboratively, with a well-defined data strategy, appropriate processes and resources, it happens/gets dealt anyway as a series of mappings/fixes/hard-coded values within ETL or BI scripts, source systems, or spreadsheets that inevitably end up presenting incomplete/misleading/conflicting truths to the decision makers. The perceived costs of such fixes may not be higher, but the business value is invariably inferior and lost opportunities typically run up to millions of dollars. Unfortunately, this is not as obvious to business leaders as it should be.
So how can we encourage higher numbers of business leaders to wake up and smell the coffee? This question is already racking the brains of many MDM marketing executives. Perhaps they can help make the leap of faith easier by reducing the entry-level costs and by taking a more flexible and collaborative approach to reduce/manage the initial project risks. There are already players in data management space who seem to be doing this in some form or another and translating it to remarkable growth.
Yasemin is a data and analytics leader with 15+ years of experience specialising in Data Modelling, Master Data Management, Data Governance and Data Warehousing. Prior to Farfetch, Yasemin worked as a Principal or Independent Consultant leading MDM and Data Warehousing projects for companies such as J&J, BP, Shell, ITG, XL Catlin, Celgene, P&G and Royal Mail. She played a leading role in the design and evolution of the Kalido MDM application, now known as Magnitude MDM. She also played a key role in several organisational initiatives for current state assessment and future state design for data strategy. Yasemin holds a PhD in Information Science from City University, London.
Copyright Yasemin Kural, Principal Data Architect, Farfetch